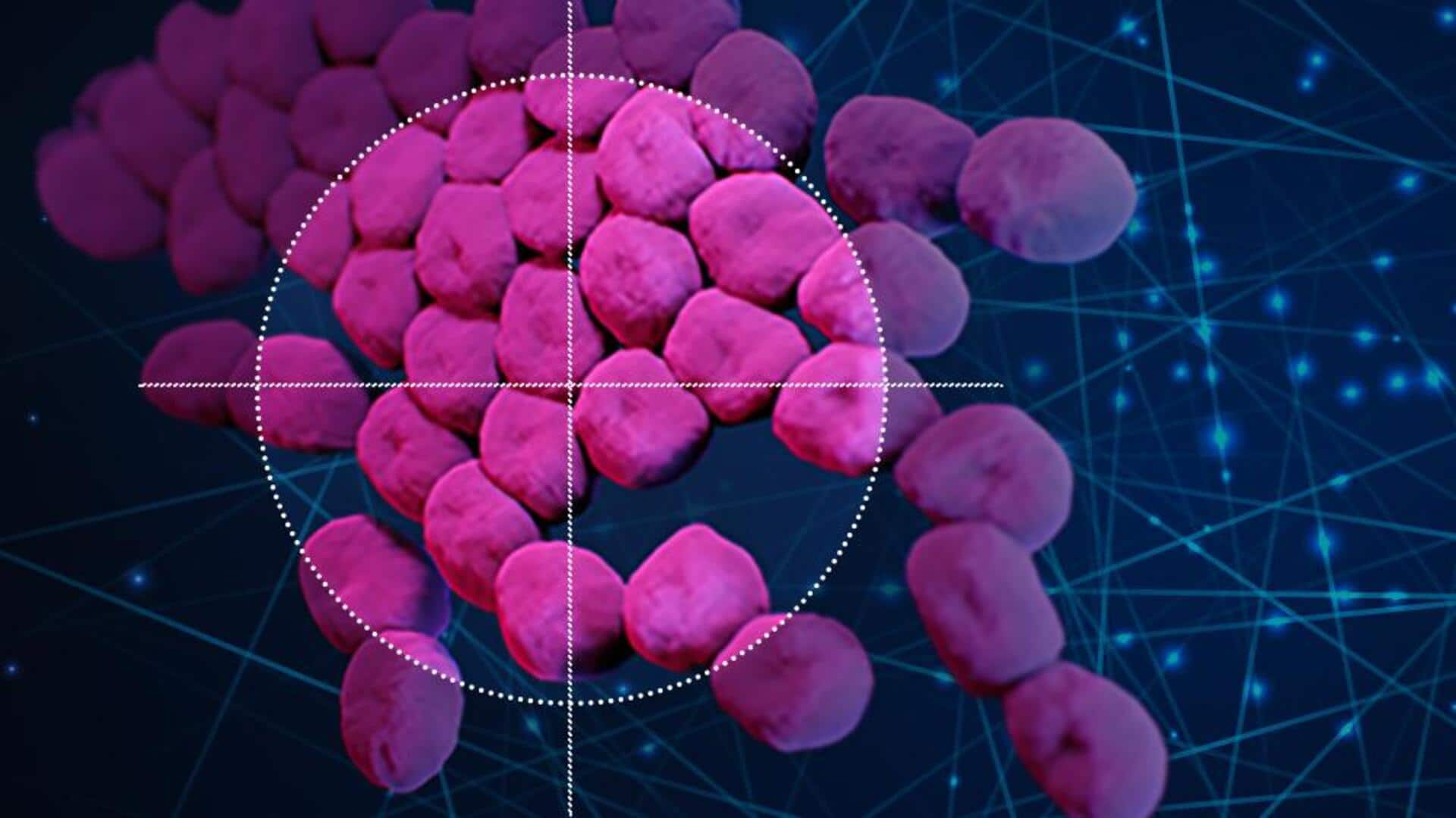
How AI is being used to detect drug-resistant infections
What's the story
Researchers at Tulane University, US have developed an artificial intelligence (AI)-based method to improve detection of genetic markers of antibiotic resistance in Mycobacterium tuberculosis and Staphylococcus aureus.
The innovative technique, detailed in a study published in Nature Communications, could potentially pave the way for faster and more effective treatments for drug-resistant infections.
These infections pose a major global health crisis owing to their treatment difficulty and higher mortality rates.
Innovative approach
How does the model work?
The study presents a new Group Association Model (GAM) that employs machine learning to pinpoint genetic mutations associated with drug resistance.
Unlike traditional tools, GAM doesn't rely on existing knowledge of resistance mechanisms, making it more versatile and able to uncover previously unknown genetic alterations.
The researchers used GAM on more than 7,000 strains of Mtb and nearly 4,000 strains of S. aureus, pinpointing key mutations linked to resistance.
Improved accuracy
GAM model surpasses WHO's resistance database
Notably, the GAM model not only matched but also surpassed the accuracy of the World Health Organization's (WHO) resistance database.
It drastically reduced false positives, which are wrongly identified markers of resistance that could lead to inappropriate treatment.
"Our method provides a clearer picture of which mutations actually cause resistance, reducing misdiagnoses and unnecessary changes to treatment," said Julian Saliba, lead author of the study and graduate student at Tulane University.
Clinical validation
AI model outperforms WHO-based methods in predicting resistance
In clinical trials with samples from China, the machine-learning-enhanced model outperformed WHO-based methods in predicting resistance to key front-line antibiotics.
This early detection capability could help doctors choose the correct treatment regimen before an infection spreads or worsens.
Notably, the model's potential application goes beyond bacteria detection and might even be used in agriculture, where antibiotic resistance is a growing concern.