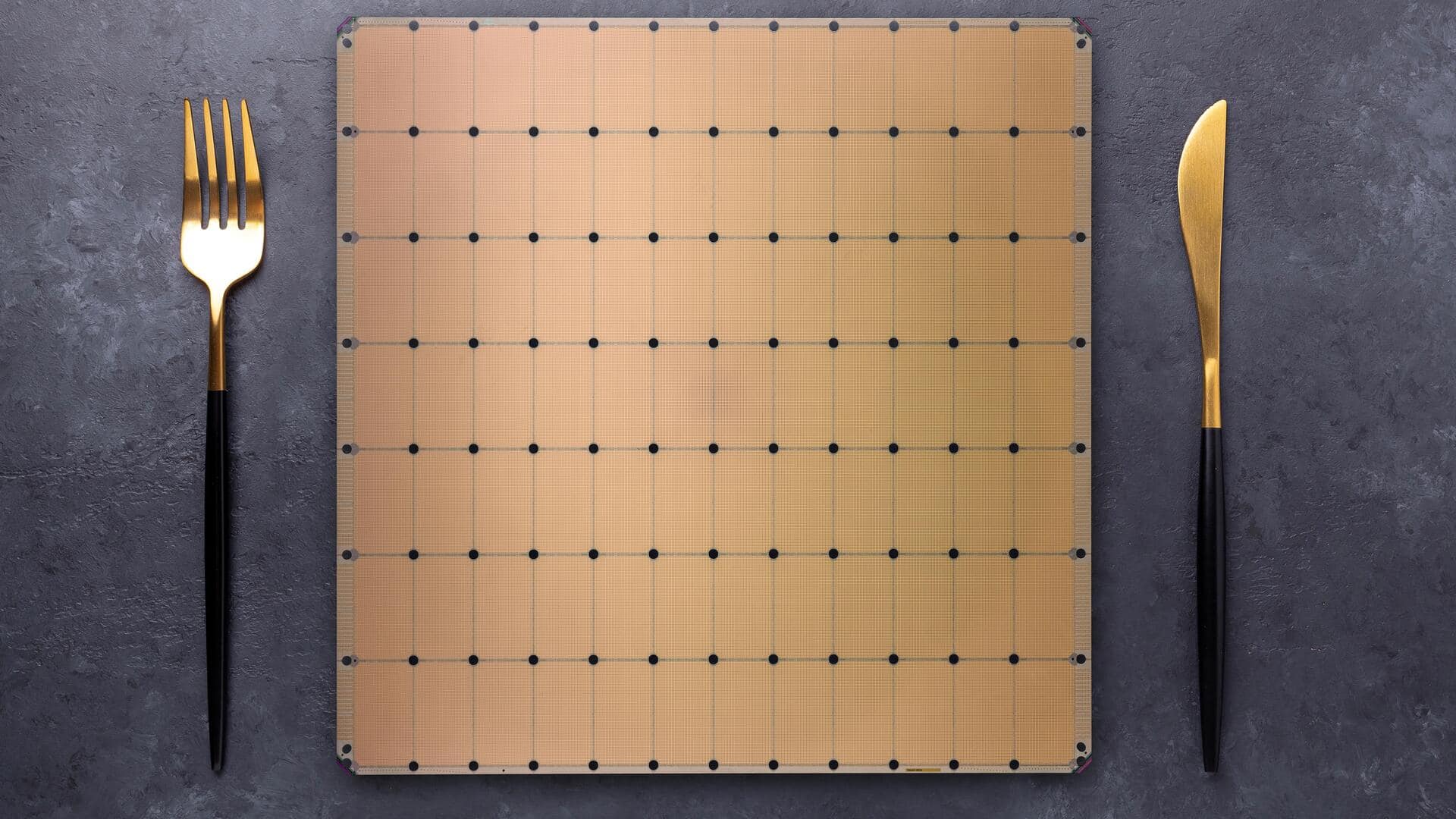
AI start-up Cerebras unveils WSE-3, the world's largest semiconductor
What's the story
US-based Cerebras Systems has introduced its third-generation AI chip, the Wafer Scale Engine 3 (WSE-3). It is touted as the world's largest semiconductor.
The WSE-3 is designed to train AI models by fine-tuning their neural weights or parameters.
The new chip doubles the performance of its predecessor, WSE-2, which was released in 2021.
However, it maintains the same power draw and price despite increased performance.
Details
WSE-3: Doubling performance and shrinking transistors
The WSE-3 chip, the size of a 12-inch wafer, has doubled its rate of instructions from 62.5 petaFLOPs to 125 petaFLOPs.
The chip's transistors have been reduced from seven nanometers to five nanometers, increasing the transistor count from 2.6 trillion in WSE-2 to four trillion in WSE-3.
The on-chip SRAM memory content has slightly increased from 40GB to 44GB, and the number of computing cores has increased from 850,000 to 900,000.
In comparison
WSE-3 outperforms NVIDIA's H100 GPU
The WSE-3 chip is 57 times larger than NVIDIA's H100 GPU, with 52 times more cores and 800 times more on-chip memory.
It also boasts 7,000 times more memory bandwidth and over 3,700 times more fabric bandwidth.
According to Cerebras co-founder and CEO Andrew Feldman, these factors underpin the chip's superior performance.
The CEO further stated that the WSE-3 can handle a theoretical large language model (LLM) of 24 trillion parameters on a single machine.
Ease of working
WSE-3: Easier programming and faster training times
Feldman argued that the WSE-3 is easier to program than a GPU, requiring significantly fewer lines of code.
He also compared training times by cluster size, stating that a cluster of 2,048 CS-3s could train Meta's 70-billion-parameter Llama 2 large language model 30 times faster than Meta's AI training cluster.
This efficiency allows enterprises to access the same compute power as hyperscalers but at a much faster rate.
Partnership with Qualcomm
Cerebras partners with Qualcomm to reduce inference costs
Cerebras has partnered with chip giant Qualcomm to use its AI 100 processor for the inference process in generative AI.
The partnership aims to reduce the cost of making predictions on live traffic, which scales with the parameter count.
Four techniques are applied to decrease inference costs, including sparsity, speculative decoding, output conversion into MX6, and network architecture search.
These approaches increase the number of tokens processed per dollar spent by an order of magnitude.
Demand
Cerebras's high demand and future inference market focus
Cerebras is experiencing high demand for its new chip, with a significant backlog of orders across enterprise, government, and international clouds.
Feldman also highlighted the future focus on the inference market as it moves from data centers to more edge devices.
He believes that easy inference will increasingly go to the edge where Qualcomm has a real advantage.
This shift could potentially change the dynamics of the AI arms race in favor of energy-constrained devices like mobiles.