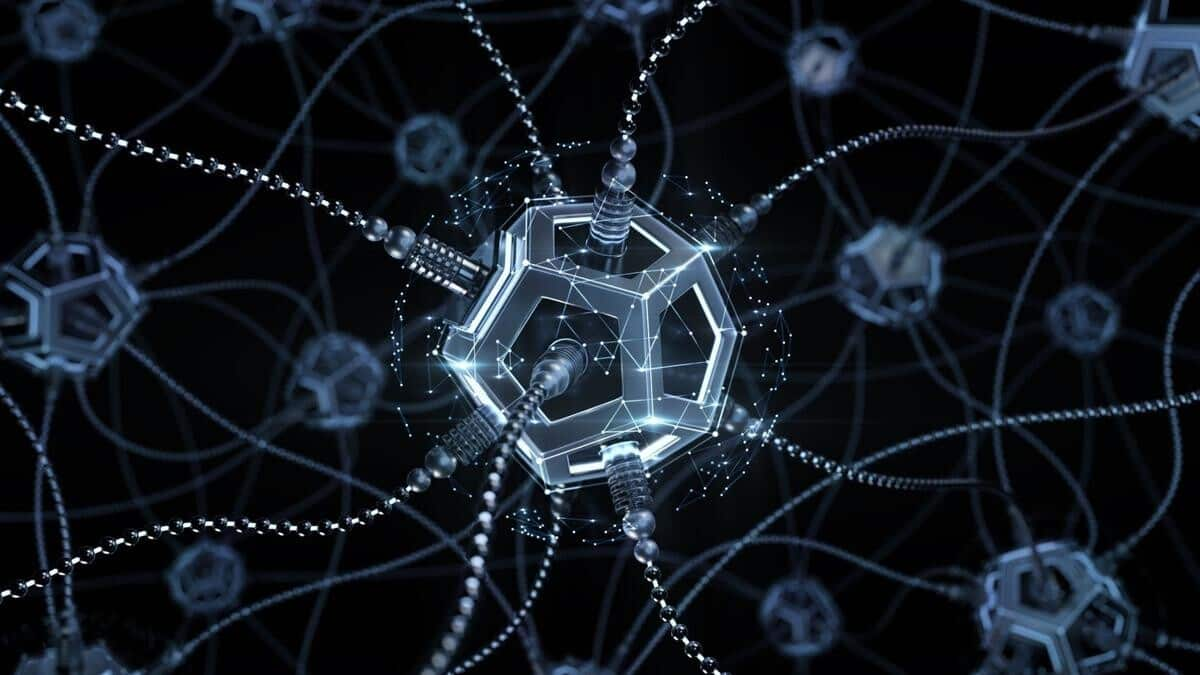
This AI model could aid in lowering carbon emissions worldwide
What's the story
Engineers at the Massachusetts Institute of Technology (MIT), have developed a machine-learning framework that can predict heat movement in semiconductors and insulators, with unprecedented speed and accuracy. This technological breakthrough could revolutionize energy generation systems, potentially reducing carbon emissions and conserving energy. The new framework, as described in a paper published in Nature Computational Science, predicts this movement or phonon dispersion relations (PDRs), up to one million times faster than traditional methods, and 1,000 times quicker than existing AI techniques.
Requirement
Why is the model necessary?
PDRs describe the relationship between energy and momentum of phonons within a material's crystal structure. "Phonons are the culprit for thermal loss, yet obtaining their properties is notoriously challenging, either computationally or experimentally," said Mingda Li, an Associate Professor of Nuclear Science and Engineering at MIT. The innovation addresses a significant global issue, as approximately 70% of generated energy is currently lost as waste heat in worldwide energy systems.
Innovative framework
MIT's novel approach to predicting phonon behaviors
To tackle the high-dimensional nature of PDRs, the researchers developed a virtual node graph neural network (VGNN). Traditional ML models struggle with this complexity. The VGNN brings flexible virtual nodes to the fixed crystal structure, enabling efficient prediction of phonon behaviors. "The way we do this is very efficient in coding. You just generate a few more nodes in your GNN," explained Abhijatmedhi Chotrattanapituk, a graduate student at MIT and co-author of the paper.
Future prospects
AI accelerates discovery of superior materials
The VGNN can quickly estimate phonon dispersion relations, and offers greater accuracy in predicting a material's heat capacity. This efficiency allows calculation of PDRs for thousands of materials within seconds on a personal computer, possibly accelerating the discovery of materials with superior thermal properties. The researchers plan to refine their technique, improving virtual nodes' sensitivity to capture minute changes affecting phonon structures.