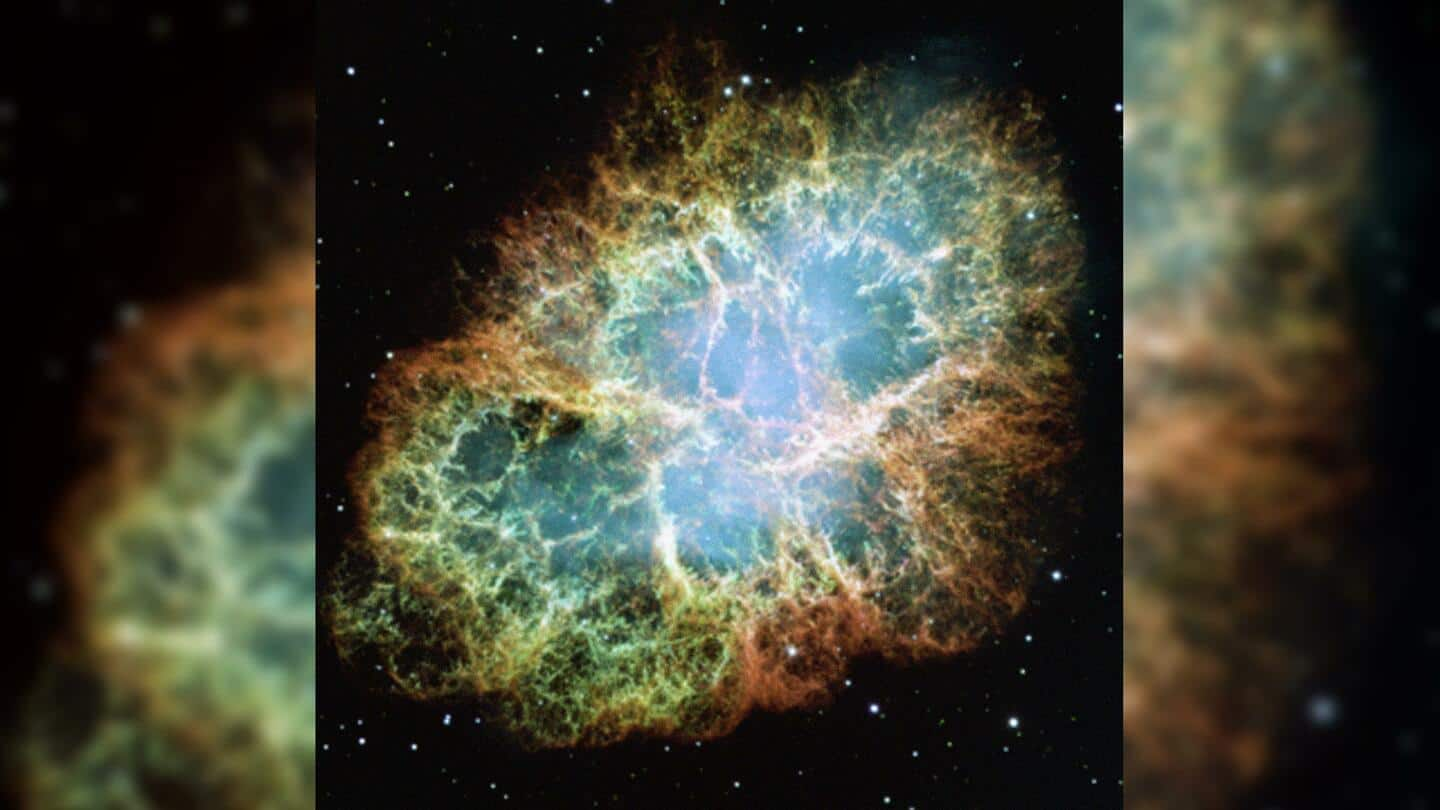
Caltech uses machine learning to classify dying stars: Here's how
What's the story
SNIascore, a machine-learning algorithm developed by astronomers from the California Institute of Technology (Caltech), has accurately classified 1,000 supernovas. They are bright and powerful explosions caused by dying stars.
The algorithm was created using data from the Zwicky Transient Facility (ZTF). It is a sky survey instrument attached to the Samuel Oschin Telescope at Caltech's Palomar Observatory near San Diego.
Context
Why does this story matter?
Every night, the ZTF scans the night sky for what's called transient events, which include blazing asteroids, devouring black holes, and supernovae.
It then sends out alerts to astronomers across the globe who carry out follow-up investigations.
Categorizing the data, that is collected every single night, is no easy task and that's where machine learning tools come into the picture.
Supernovae
Supernovae are of 2 types
Supernovae fall under two broad categories- Type 1 and Type 2, the former being more common.
Type I occurs when matter flows from a companion star to a white dwarf star, resulting in a thermonuclear explosion, and Type II takes place when a massive star collapses under its own gravity.
Type I supernovae are devoid of hydrogen, whereas Type II are abundant in hydrogen.
Prowess
SNIascore can only classify Type 1a supernovae
Currently, the SNIascore algorithm can classify Type 1a supernovae, a sub-group of Type 1 cosmic explosions.
They are also called 'standard candles' because the explosion from these dying stars results in a uniform light output and can help estimate the universe's expansion rate.
Caltech astronomers are working on improving this algorithm to detect other types of supernovae in the future.
Process
How does SNIascore work?
The data collected by ZTF each night is transferred to an instrument called Spectral Energy Distribution Machine (SEDM) at Palomar.
SNIascore, along with SEDM, categorizes the observed supernovas and picks out whichever fits under the Type 1a class.
This "reliable data set of supernovae" can be used further to investigate the physics of such powerful stellar explosions.
Official words
SNIascore was launched on April 2021
"SNIascore is remarkably accurate," said Christoffer Fremling, a Caltech astronomer and the mastermind behind the algorithm.
"After 1,000 supernovae, we have seen how the algorithm performs in the real world," he added.
"We have found no clearly misclassified events since launching back in April 2021, and we are now planning to implement the same algorithm with other observing facilities," Fremling claimed.
Information
Machine learning tools can help identify habitable planets
Astronomers firmly believe that machine learning tools will help them identify which other planets might be habitable. For instance, Morpheus, an AI developed by researchers at the University of California, can detect and classify galaxies based on raw data from telescopes.