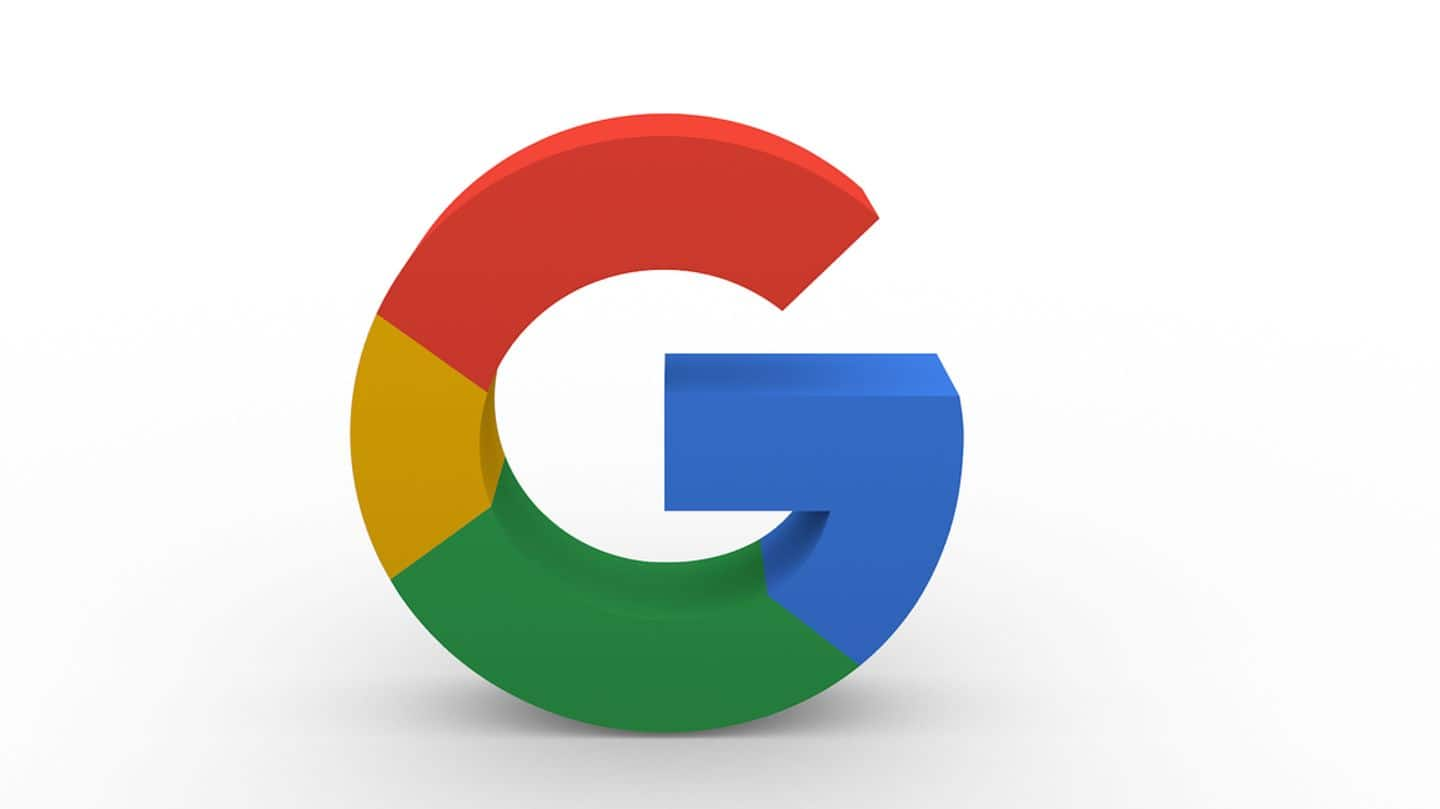
Google's AI detects breast cancer with 99% accuracy: Details here
What's the story
Google has developed an AI that can detect advanced breast cancer much more effectively than pathologists. The deep learning tool, as the company describes, can detect cancers with as much as 99% accuracy. The findings were made in two separate studies designed to assess the performance and efficiency of the tool. Here are the finer details.
The tool
Google's LYmph Node Assistant, or LYNA tool
Last year, researchers at Google described a deep learning-based approach for improving advanced breast cancer diagnosis. The approach, dubbed LYmph Node Assistant or LYNA, was used to create algorithms, which were fed with data from cancer patients and was transformed into a tool. Now, that tool has been put to test to see how it could improve the diagnosis process.
Test 1
Detection between cancerous and non-cancerous samples
In the first test, the tool was used to screen two different sets of pathology slides - one that had already been used for training and other were independent samples from a different lab. However, despite the element of diversity, LYNA was successful in identifying tumor characteristics. In both the cases, the tool correctly distinguished between cancerous and non-cancerous slides 99% of the time.
Do you know?
It even detected smallest of the metastases
More interestingly, along with location and characteristics of the main tumor, the tool also revealed other suspicious metastases, including those that were too small to be detected by pathologists. This, as the company said, could easily reveal all areas of concern for better diagnosis and treatment.
Test 2
In the second test, LYNA increased detection speed
In the second test, the researchers assigned six certified pathologists to conduct simulated diagnoses with and without LYNA. They looked at lymph nodes for metastatic breast cancer and found the AI engine not just made their work simpler but faster too. It reduced the rate of missing micrometastases by a factor of two and halved the analysis time to one minute per slide.
Future prospects
The technology is promising, but still needs work
While the accuracy of this deep learning tool is promising, the researchers have stressed the importance of its evolution. Right now, it has some limitations and has not entered real clinical workflows, which would be critical to assess the further use and efficiency of the technology. Notably, it could even be adapted for other forms of tumors, leading to more effective diagnosis and treatment.