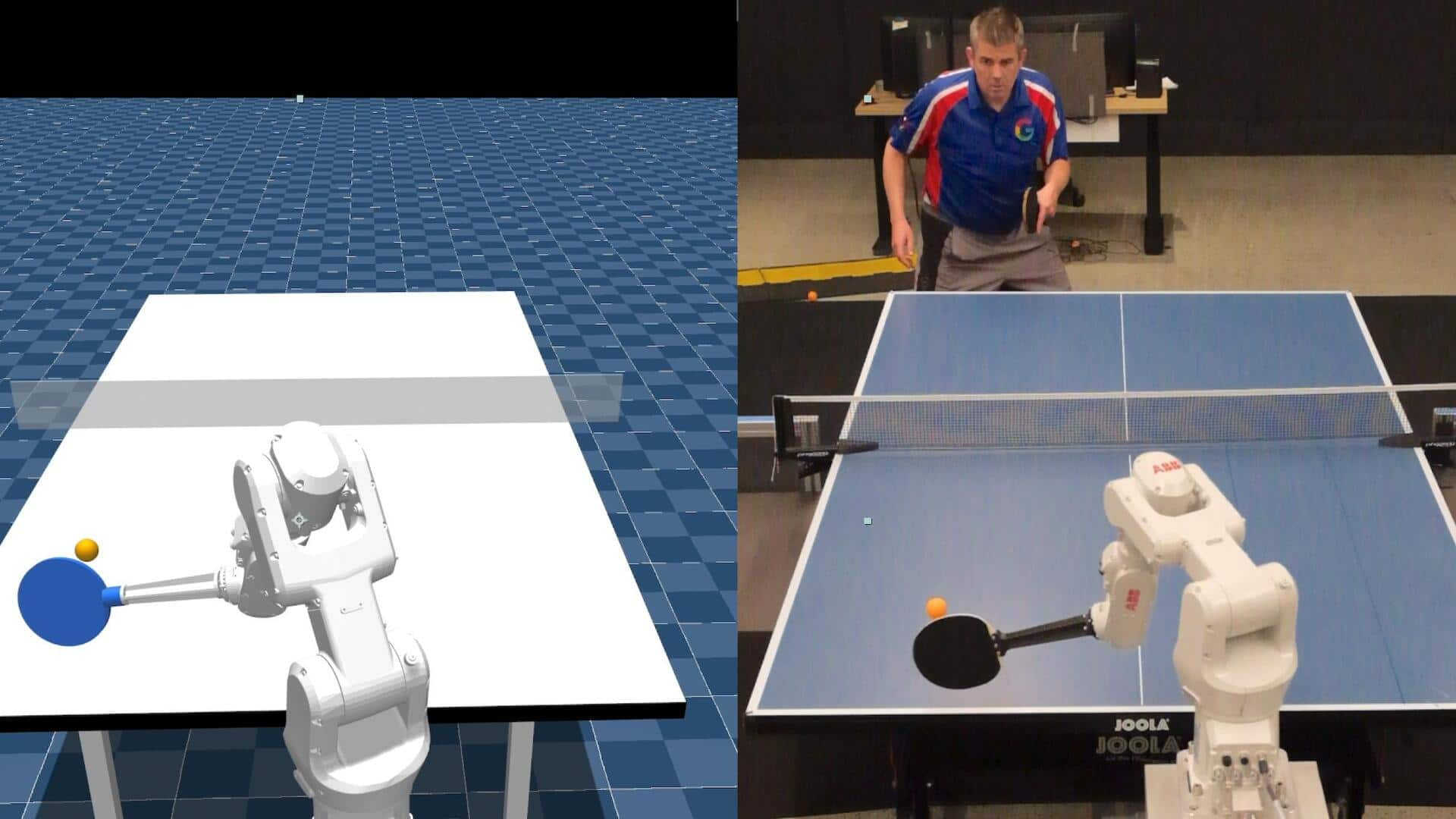
Google's new robot can play table tennis like humans
What's the story
Google's DeepMind team has made a significant breakthrough by developing a table tennis-playing robot.
This robot can compete at an amateur human level, as detailed in Google's recently published paper "Achieving Human Level Competitive Robot Table Tennis."
The research paper asserts that this is the first-ever robot capable of matching humans in a sport, marking a major advancement in robotic learning and control.
Twitter Post
Watch the robot playing
Robotic table tennis has served as a benchmark for this type of research since the 1980s.
— Google DeepMind (@GoogleDeepMind) August 8, 2024
The robot has to be good at low level skills, such as returning the ball, as well as high level skills, like strategizing and long-term planning to achieve a goal. pic.twitter.com/IX7VuDyC4J
Competitive edge
Performance against human opponents
The table tennis robot was put to the test against beginner and intermediate-level players.
It demonstrated its prowess by defeating all beginner-level opponents and securing victories in 55% of matches against intermediate players.
However, the robot fell short when pitted against advanced players, losing all matches.
Out of the 29 games it participated in, the system emerged victorious in 45% of them.
Research standard
Table tennis: A benchmark for robotic research
The sport of table tennis has long been a standard for robotic research since the 1980s.
This is due to its demand for both basic skills like returning the ball, and complex ones such as strategizing and long-term planning.
Despite their success, DeepMind's team acknowledges that more work is needed to consistently achieve human-level performance on single tasks, and eventually develop generalist robots capable of performing various useful tasks.
Room for improvement
Challenges and potential improvements in robotic performance
The table tennis robot's primary weakness is its slow reaction time to fast balls.
This issue is attributed to system latency, compulsory resets between shots, and a lack of useful data.
To overcome these challenges, the researchers suggest exploring advanced control algorithms and hardware optimizations.
These could include models for predicting ball trajectories or implementing faster communication protocols between the robot's sensors and actuators.
Broader implications
DeepMind's research could impact broader robotics
Beyond table tennis, DeepMind envisions that their research could have wider implications for the field of robotics.
This could be through policy architecture, the use of simulation in real games, and real-time strategy adaptation.
The team also identified areas for improvement such as handling high and low balls, backhand shots, and deciphering the spin on an incoming ball.