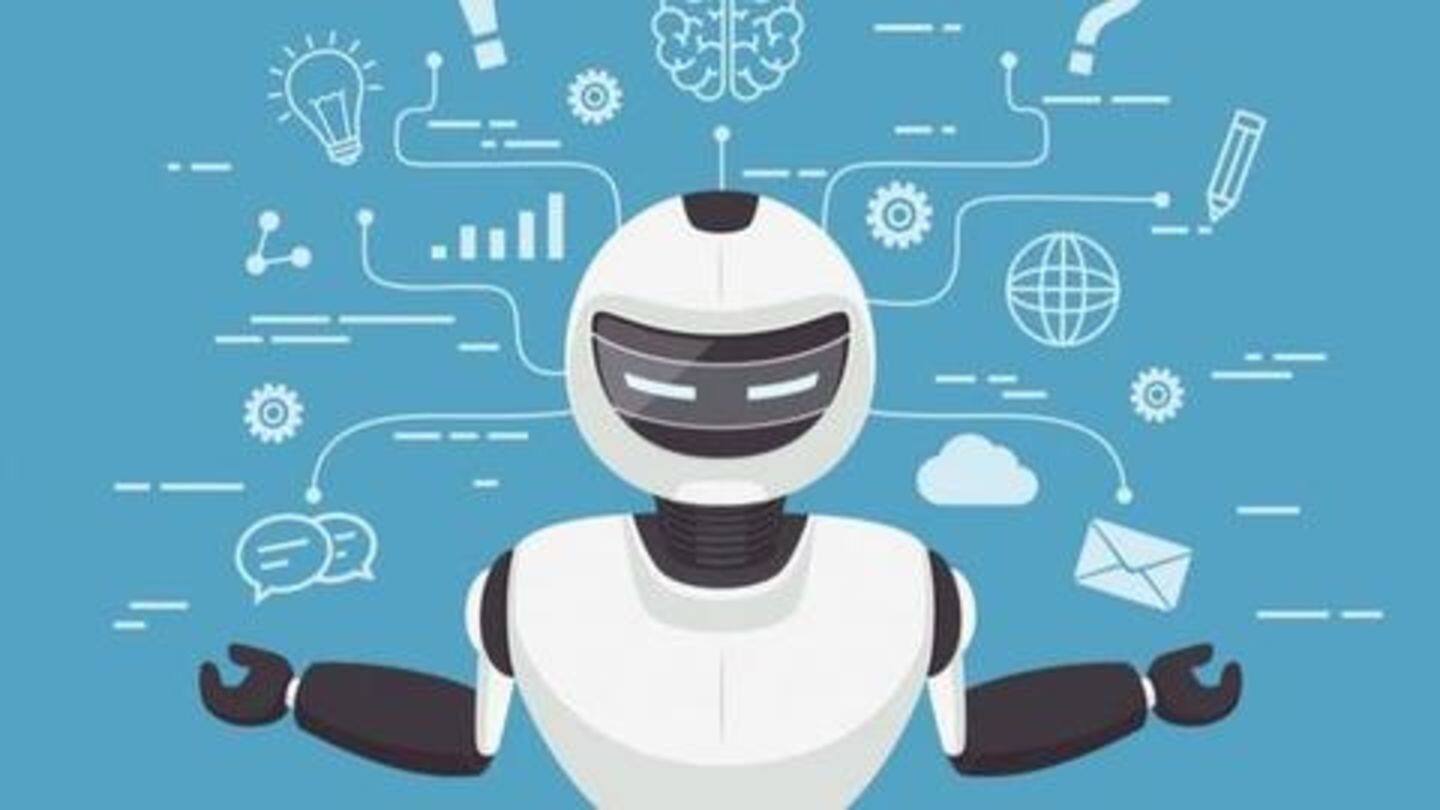
AI can predict a lot, but it's not a movie-buff
What's the story
Artificial intelligence (AI) and machine learning (ML) is increasingly making their way into almost every aspect of our lives, and the movie industry is no exception. Recently, movie studio 20th Century Fox developed a machine learning algorithm to help itself predict which movies would be a hit with particular audiences based on an analysis of movie trailers. Yet, the findings were far from perfect.
Premise
The premise on which the machine learning algorithm works
The machine learning-based analysis system, described in a paper published in late October, is essentially an image detecting algorithm that analyzes and categorizes movie trailers based on the objects and people it identifies in the trailer. The premise is that if an audience, 'A', enjoys a trailer of a movie, 'X', audience 'A' will also enjoy movies 'Y' and 'Z', provided that trailers for 'Y' and 'Z' are similar to the trailer of 'X'.
Analysis
How the algorithm analyzes movie trailers
The algorithm examines a movie trailer frame by frame, and then labels objects, people, and events in the trailer based on the frequency at which they appear on screen, and based on the duration for which they remain on screen. This allows the algorithm to categorize trailers based on the trailer's elements, and is helpful as it correlates with a movie's genre.
Quote
An illustration of how the algorithm works
"For example, a trailer with a long close-up shot of a character is more likely for a drama movie than for an action movie, whereas a trailer with quick but frequent shots is more likely for an action movie," wrote 20th Century Fox engineers.
Testing
The algorithm was tested on the trailer of 'Logan'
To test the algorithm, researchers tested it on the trailer of superhero movie 'Logan', and tried to predict which movies the audience of 'Logan' would enjoy. The algorithm analyzed the trailer of 'Logan', and concluded that the most recurring elements were "trees", "facial hair", "car", "man", and "vehicle", respectively. Based on 10 most recurring elements, it then made its predictions.
Predictions
Impressively, the algorithm made several correct predictions
The algorithm's predictions were then correlated with actual data on the top 20 films people who went to watch 'Logan' also watched. Impressively, the algorithm got 11 out of 20 predictions correct, including the top five films these people actually watched. However, the wrong predictions demonstrated the limitations of algorithms in matters of human subjectivity.
Correct picks
The correct predictions were made on objective criteria
Based on the aforementioned elements, the algorithm correctly identified that 'Logan' watchers would not just enjoy watching superhero films like 'Dr. Strange', 'Batman vs Superman: Dawn of Justice', 'Suicide Squad', 'X-Men: Apocalypse' etc., but would also enjoy action flicks like 'John Wick 2, 'Terminator: Genisys', and 'The Magnificent Seven'. In all these cases, the algorithm's analytic objectivity helped it identify similar trailer elements correctly.
Wrong picks
The algorithm, however, failed the interpretation test
The algorithm wrongly predicted that 'Logan' watchers would also enjoy watching 'The Revenant' and 'Tarzan', presumably because these movie trailers have an abundance of "trees" and "facial hair". Yet, the algorithm failed to predict that 'Logan' watchers might enjoy 'Deadpool' and 'Ant-Man', both of which are not just Marvel movies, but also portray their protagonists in an off-beat way that might have been appealing to fans of 'Logan'. Objectivity, thus, failed the test of interpretation.
Subjectivity
Again, AI falls short of understanding human subjectivity
What this essentially demonstrated (perhaps for the Nth time) was that artificial intelligence (AI) is still far from accurately understanding matters of human subjectivity. While AI can do wonders in finding objective details and patterns that might escape us humans, it simply cannot, as of now, understand how humans process information and react to stimuli.