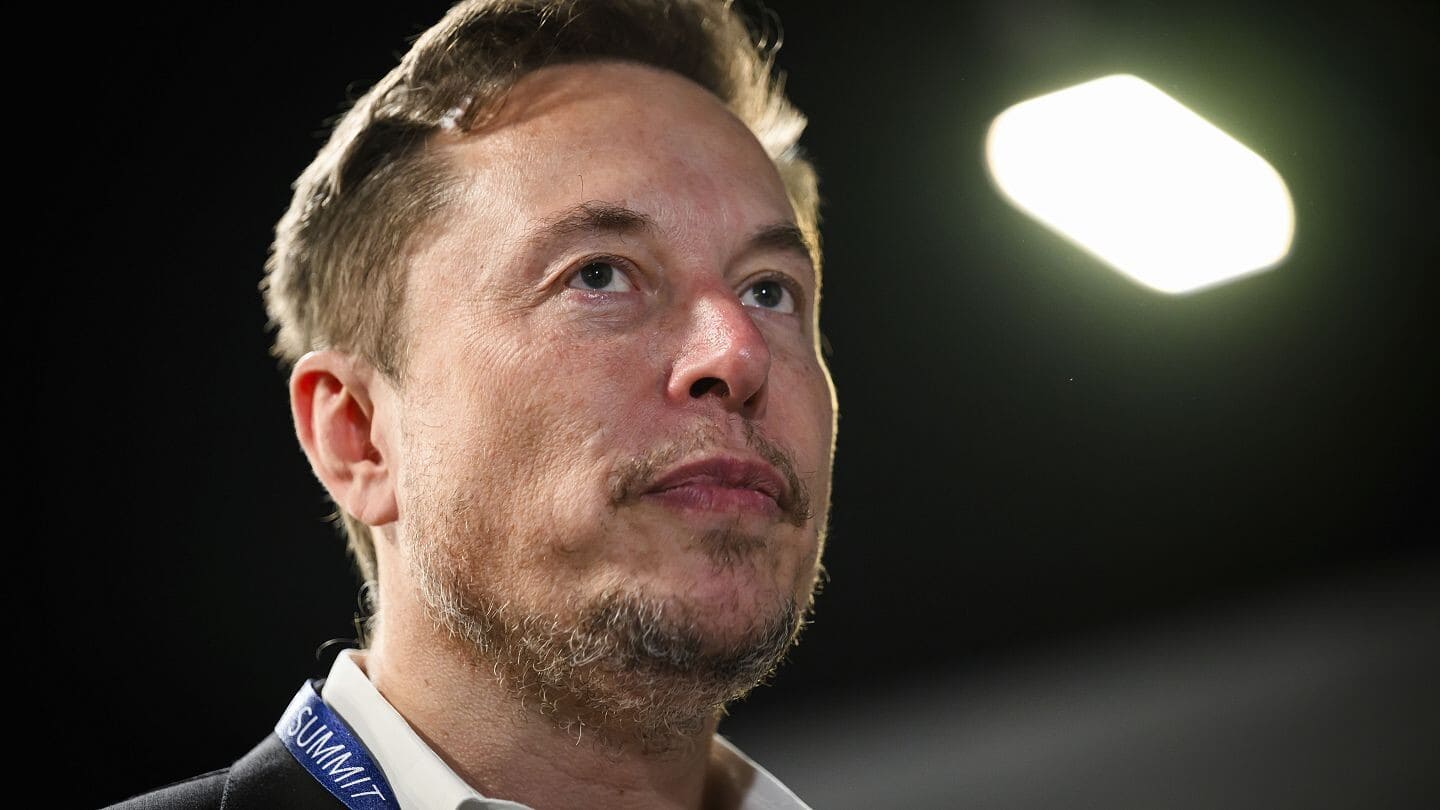
Real-world data for AI training exhausted? Elon Musk thinks so
What's the story
Elon Musk, owner of AI firm xAI, has agreed with other AI experts that the pool of real-world data to train AI models is almost empty. Speaking during a live-streamed discussion with Stagwell Chairman Mark Penn, Musk said, "We've now exhausted basically the cumulative sum of human knowledge... in AI training. That happened basically last year." This comes in line with former OpenAI Chief Scientist Ilya Sutskever's claim at NeurIPS, a machine learning (ML) conference last December.
New direction
What is the future of AI training?
Sutskever had hinted that the AI industry has hit a point of "peak data," and this scarcity will require a change in the way models are being developed today. Musk supported this notion, offering synthetic data—information generated by AI models themselves—as the answer. He said, "The only way to supplement [real-world data] is with synthetic data, where the AI creates [training data]. With synthetic data ... [AI] will sort of grade itself and go through this process of self-learning."
Industry shift
Tech giants turn to synthetic data for AI training
Several tech giants, including Meta, Microsoft, OpenAI, and Anthropic, are already using synthetic data to train their flagship AI models. According to Gartner's research, 60% of the data used for AI and analytics projects in 2024 were synthetically generated. This trend is already visible in Microsoft's Phi-4 and Google's Gemma models, both trained on a combination of real-world and synthetic data.
Cost and risk
Synthetic data: A cost-effective alternative with potential risks
The use of synthetic data also comes with financial benefits. AI start-up Writer claimed that its Palmyra X 004 model, trained mostly on synthetic sources, only cost $700,000 to develop. That's way lower than the estimated $4.6 million for a similarly-sized OpenAI model. However, researches suggest potential risks of synthetic data like model collapse—where a model's outputs become less "creative" and more biased over time due to the inherent biases and limitations in the training data used by these models.