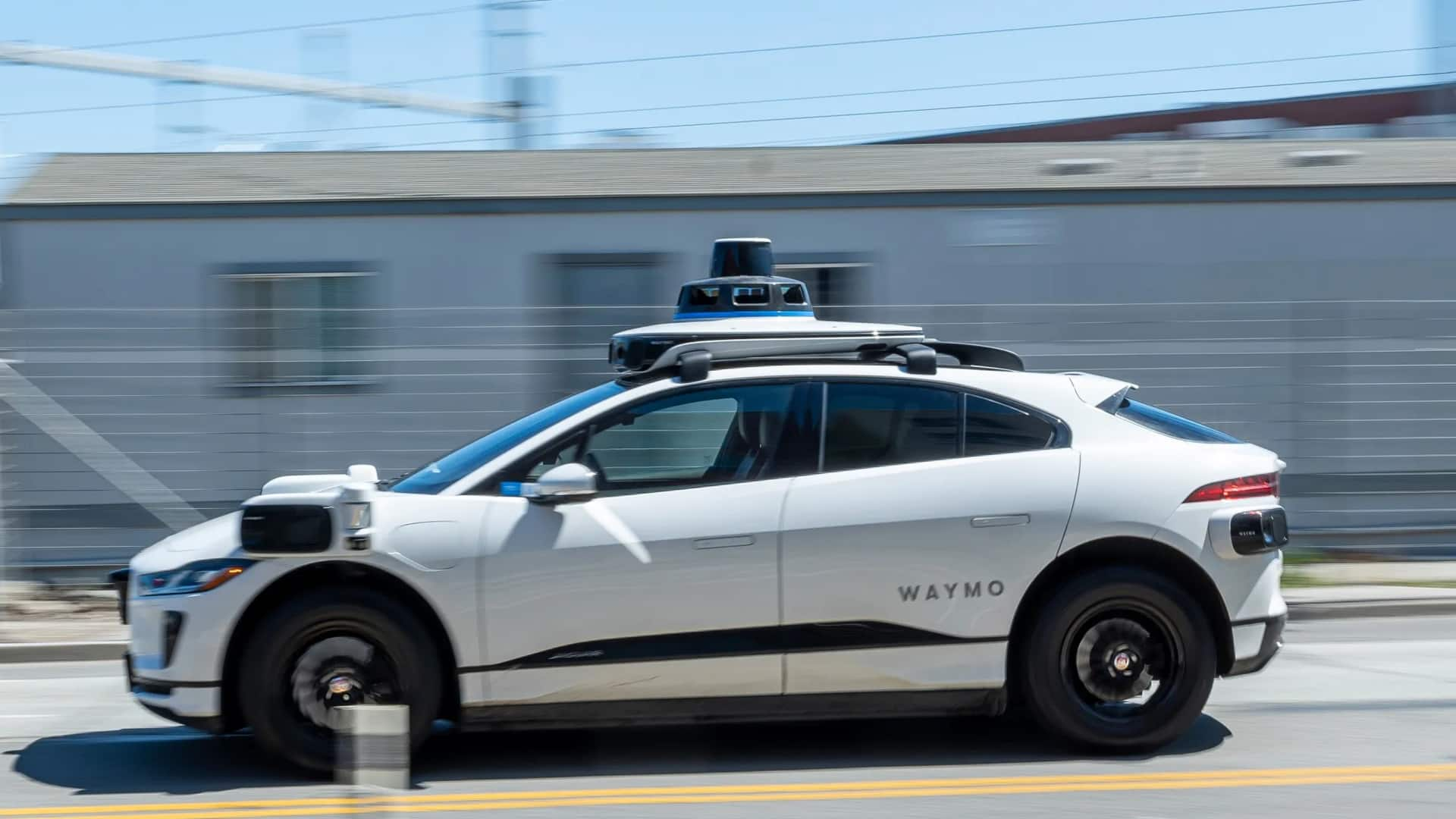
Gemini AI to train Waymo's self-driving taxis: Here's how
What's the story
Waymo, a frontrunner in the autonomous driving arena, is capitalizing on its partnership with Google's DeepMind to stay ahead of the competition. The company has now announced plans to utilize Google's multimodal large language model (MLLM) Gemini, for training its self-driving cars. The technique, disclosed by Waymo in a research paper, is referred to as "End-to-End Multimodal Model for Autonomous Driving," or EMMA.
Model details
EMMA: A new training model for autonomous vehicles
The newly introduced EMMA is a holistic training model that leverages sensor data to predict future paths for self-driving cars. This advanced model helps Waymo's autonomous vehicles with decision-making processes like route selection and obstacle avoidance. The use of MLLMs like Gemini in an entirely new environment (on the road) is a major departure from their applications in chatbots, email organizers, and image generators.
System improvements
Overcoming challenges in autonomous driving systems
Traditional autonomous driving systems have depended on dedicated modules for tasks like perception, mapping, prediction, and planning. However, this approach has struggled with scalability problems due to compounded errors and restricted inter-module communication. MLLMs like Gemini present a possible solution to these problems with their extensive training data sets and advanced reasoning capabilities that emulate human thought processes.
Model evaluation
EMMA's performance and future prospects
Waymo has claimed its EMMA model has excelled in complex scenarios like encountering animals or construction on the road. The company said, "This suggests a promising avenue of future research, where even more core autonomous driving tasks could be combined in a similar, scaled-up setup." However, Waymo also noted that more research is required before fully deploying this model owing to certain limitations.
Model limitations
Limitations and risks of using MLLMs in autonomous driving
Despite its potential, EMMA has its limitations. For instance, it cannot take 3D sensor inputs from LiDAR or radar due to high computational costs. It can also only handle a limited number of image frames at a time. Further, there are risks involved in using MLLMs like Gemini for training self-driving cars as they often hallucinate or fail at simple tasks like reading clocks or counting objects.